VISUALIZING DATA FOR MEANINGFUL IMPACT ON DECISION MAKING
Based on tips from Anneli Joplin
Volume 2 Issue 4 (2020), pp. 15-16
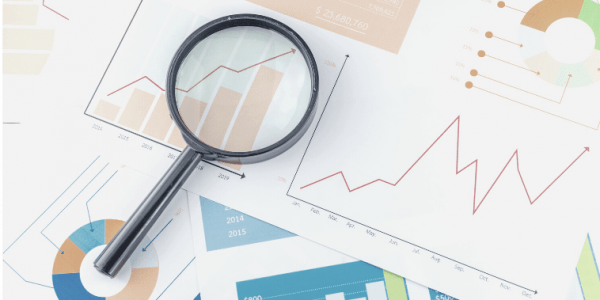
What makes for impactful data visualizations? How do those visualizations help facilitate effective dialogue with practice-side partners about research findings that affect decision making?
These are important questions for many of us who work in research-practice partnerships (RPPs). At this year’s NNERPP Annual Forum, our annual conference which brings together our members, funders, and relevant policy and advocacy groups, we were joined by data viz expert Anneli Joplin for a highly popular, much praised session that addressed these exact questions. During the session, Anneli shared many practical tips and concrete examples with Annual Forum participants. There was so much interest in the topic within the NNERPP community that following Anneli’s session, we launched a NNERPP Data Viz Club, an informal club for NNERPP members to share, discuss, and receive feedback on data visualizations in monthly virtual meetings.
In order to keep these conversations supported, we share with you this edition of “Extra Credit”, where we present three main takeaways from Anneli’s presentation that we hope are beneficial to those working in RPPs, or those in the broader education field that want to learn more about designing meaningful data visualizations.
Before we start, a bit more about Anneli: Anneli earned her PhD in chemistry at Rice University, at which time she discovered that her favorite part of chemistry research was visually expressing complex ideas. She has been teaching these skills since then. Following her PhD, she worked as Instructor of Visual Communication & Design at Rice University, and now is an Instructional Designer at Camden Property Trust. You can learn more about her here and find her on Twitter here: @AnomalyJoplin.
Throughout Anneli’s presentation at the NNERPP Annual Forum, the following three themes stood out; these might be particularly helpful for those seeking to design better and more useful data visualizations:
1. Understand how your viewer absorbs information.
Data visualizations are effective when designers take into account how human memory and information processing functions. Your viewer will better and more quickly understand the main idea behind your data visualization and is more likely to act on that information later if you have designed it in a way that is conducive to how the human brain processes and remembers verbal and visual information. The challenge is that the working memory’s capacity is actually quite limited, so your overall goal, says Anneli, should be to make its job easier. This basic approach to data visualization can make a big difference in your data visualization efforts.
>>To learn more about working memory, here is a place to start.
2. Then, make it easier for your viewer to absorb the information in your visualization.
Based on an understanding of human cognition and memory, there are several design principles that facilitate the brain’s job in processing and remembering information contained in a data visualization.
Among the principles that Anneli shared here, the following stood out to us for ease of integration (i.e., no fancy data viz programs needed!): Use horizontal text in your data visualizations, which makes it easier to process and read; reduce the distance between labels and data sets and/or between the data sets you are comparing so there’s less information for your brain to remember; and use symbols instead of labels/text in your data visualizations which can often be processed faster than text. Such symbols should be universally understood, non-ambiguous symbols. Here at NNERPP, we often use Canva to find icons/symbols.
Anneli also recommended taking advantage of “preattentive processing”: instantaneous and automatic processing, which helps the brain make sense of data visualizations much faster without taking up much of the brain’s limited processing and memory capabilities. For example, principles of pattern recognition can be especially useful for data visualizations with different groups of data or items of comparison. Of the pattern recognition principles that Anneli shared, two stood out to us as especially easy to integrate into any such data visualization: Proximity and similarity. The human brain automatically assumes that objects that are close together are grouped and that objects that share obvious visual features (such as color or shape) are grouped. These two simple principles can help you create visualizations that are easier and faster to process.
>>To read more about preattentive processing and preattentive visual properties, here is a place to start.
3. Remember: Balance is key.
Anneli shared many excellent design principles that help viewers make sense of data visualizations and better remember the information, which is important if we want our visualizations to impact decision making. However, she also cautioned against using too many of these principles at once, reminding us that balance is key. As a general rule, important information should be elevated and extraneous information should be removed or deprioritized. For example, consider removing gridlines in a graph if they are not needed for clarity, or make the data stand out more by using different weights for your graph versus your gridlines. Generally, strong visuals should be reserved for things that really matter and should add meaning instead of introducing noise. For example, when using the similarity principle of pattern recognition mentioned above, use visual cues to enforce the same grouping only (e.g. red squares and blue circles) instead of introducing multiple layers of groupings (e.g. a combination of red and blue circles as well as red and blue squares), and consider using no more than two visual cues (in this example, color and shape).
Lastly, a note about equitable data visualizations
Equity in data visualization is hugely important – and complex. There are many questions designers should ask themselves, including: “Was the data collected in an inequitable way?”, “Does this visualization obscure the root cause of this data?”, and “Does this visualization represent white groups as the norm and non-white groups as the ‘other’?”. If the answer is ‘yes’ to any of these, the data visualization is not equitable.
>>For a more in-depth examination of what equitable data visualization and data use looks like, Anneli pointed us to resources from Heather Krause, particularly her lecture “How Not To Use Data Like a Racist.”
Additional resources
The main takeaways we shared here are just a taste of Anneli’s rich knowledge and excellent tips for designing impactful data visualizations. Luckily, Anneli also left us with some excellent recommendations for additional data viz resources:
- Stephanie Evergreen, data visualization and design expert: She has a fantastic website and blog and runs the Evergreen Data Academy. You can connect with her on Twitter here: @evergreendata
- Ann K. Emery, data visualization speaker and designer: Check out Data Depict Studio, where she offers professional development through data visualization online courses, virtual workshops, on-site workshops, and conference keynotes, and her blog. You can connect with her on Twitter here: @AnnKEmery
- The Data Visualization Society, an organization dedicated to fostering a community for data visualization professionals worldwide. Membership is currently free. Explore their website here and find them on Twitter here: @DataVizSociety
Effective data visualization is a big and complex topic – even considerations such as which colors to pick and the uses, advantages, and disadvantages of different types of graphs are complex on their own. Yet, the three takeaways we share here from Anneli’s (much more in-depth) presentation are foundational for any type of graph or visualization displaying any kind of data, and we hope these present a helpful starting point. At NNERPP, we look forward to many more conversations and explorations around impactful data visualizations that help RPPs achieve their goals.
Anneli Joplin is Instructional Designer at Camden Property Trust.
Suggested citation: Visualizing Data for Meaningful Impact on Decision Making. (2020). NNERPP Extra, 2(4), 15-16.
NNERPP | EXTRA is a quarterly magazine produced by the National Network of Education Research-Practice Partnerships | nnerpp.rice.edu